Wildfires have experienced a surge in frequency and magnitude over the past decade, causing widespread damage to ecosystems and human settlements. Innovations in predictive analytics, remote sensing, and data integration enable more accurate and early warnings, mitigating wildfire disasters.
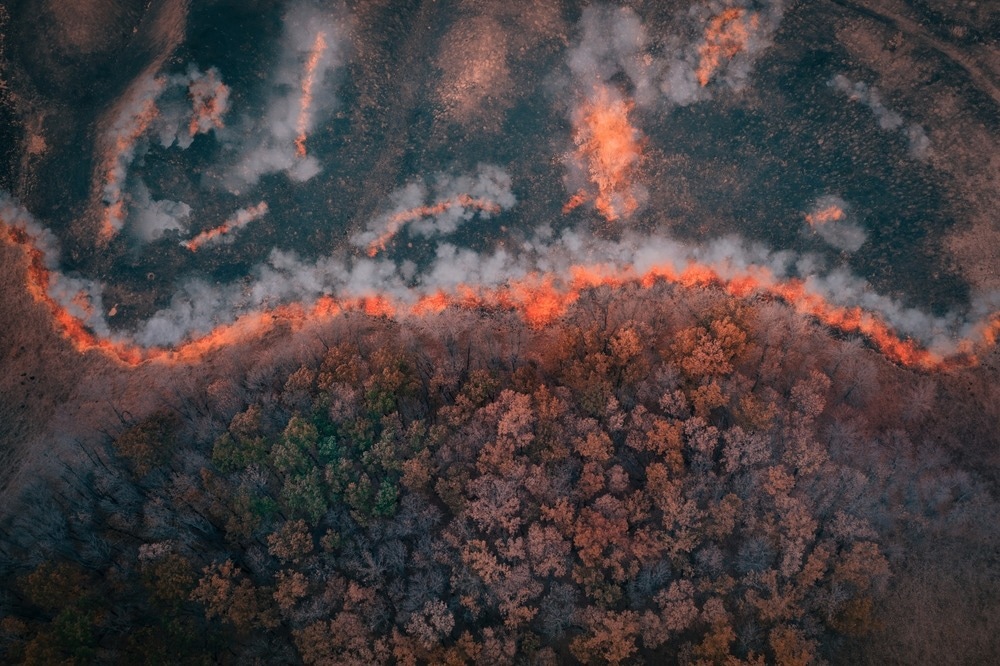
Image Credit: Che Media/Shutterstock.com
The European Space Agency estimates that wildfires have affected approximately four million square kilometers of land globally. In the United States alone, the National Interagency Fire Center reported 50 major wildfires between January 1 and June 29 last year, surpassing the 10-year average and burning approximately 192,016 acres.1
While complete prevention is impossible, the potential devastation caused by wildfires can be significantly reduced through early detection and precise geolocation in their initial stages.
Challenges in Wildfire Detection
Detecting wildfires during their early stages is challenging. Wildfire-prone areas often consist of remote, rugged terrains with limited connectivity and visibility.
Incipient fires at lower altitudes can go unnoticed for a significant duration, as they may produce limited observable indicators, such as small smoke plumes or subtle spikes in temperature and gases. Traditional wildland fire detection also heavily relies on the chance occurrence of witness reporting.
Therefore, automated approaches, independent of human involvement, are required for more efficient and accurate monitoring of vast and remote areas.
Recent Innovations in Early Wildfire Detection Systems
Smart Sensor Networks
Interconnected ground sensor networks provide an efficient solution to monitor wildfires over large geographic areas. These networks detect early-stage wildfires by monitoring temperature, humidity, air particles, and gases. They wirelessly alert centralized control systems before open flames or visible smoke appear.
Dryad Networks, a German startup, developed solar-powered sensors that act as "electronic noses," detecting smoke chemicals emitted during a fire's earliest stages. The sensors are trained using artificial intelligence (AI) to distinguish wildfire smoke from other sources, such as vehicles.
Approximately 400 sensors have been deployed in Germany's Brandenburg Forest, with alerts relayed to authorities within 10-15 minutes if smoke is detected—significantly faster than human responders.2 This quick notification enables timely response while fires are still small and manageable.
However, the sensors are limited by line-of-sight, so multiple distributed networks are needed for comprehensive monitoring.
Aerial Detection Systems
Aerial systems like drones and satellites provide a bird's eye view to spot developing wildfires.
In response to the high incidence of forest fires in California, UC Davis researchers proposed a novel approach to early wildfire detection using unmanned aerial vehicles (UAVs).
This method incorporates a ground-based sensor network across multiple sites, monitoring temperatures, humidity, and wind speed. Octocopter drones are deployed for inspection when these sensors detect conditions conducive to wildfires, such as hot, dry weather and strong winds.
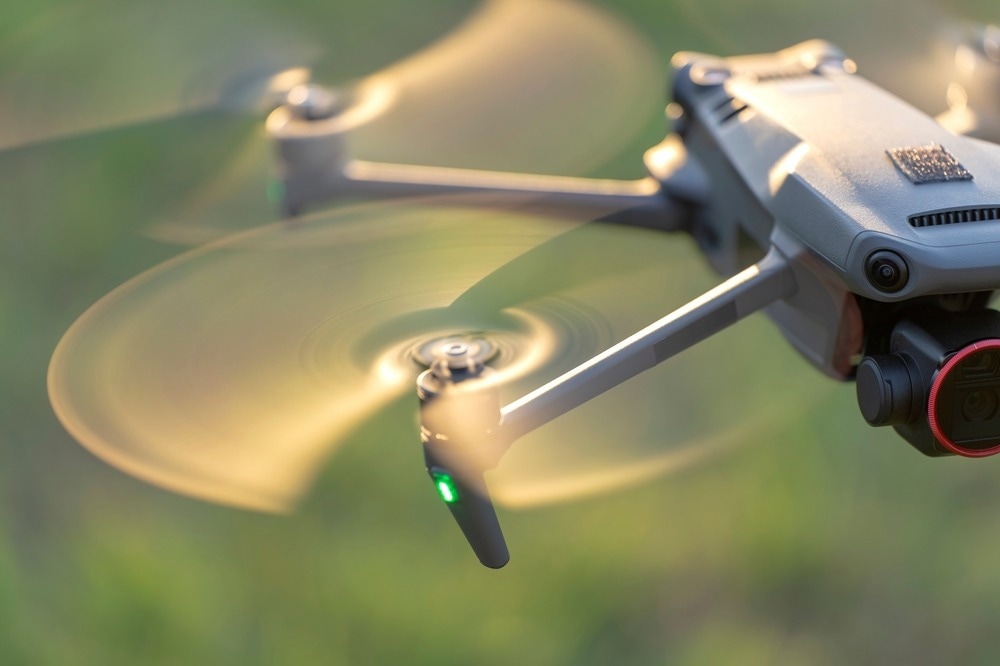
Image Credit: Bilanol/Shutterstock.com
The drones have specialized packaging tubes for collecting smoke samples and chemical sensors to provide real-time data on CO2 and smoke particles. This enables the mapping of chemical fire signatures in complex landscapes before visible detection.4
"Chemical sensing is more sensitive than visual sensing. If you walk around your neighborhood and someone's burning wood in their fireplace, you don't see anything, but you smell it," says Anthony Wexler, Director of UC Davis Air Quality Research Center.3
The mobility and range of aerial systems provide visibility over the expansive wildland-urban interface. However, weather, flight duration, and autonomy challenges remain barriers to comprehensive deployment.
AI and Computer Vision
Increasingly complex AI and computer vision algorithms are employed for automated early wildfire detection. Deep learning models are trained on thousands of fire and smoke images to recognize high-reliability visual indicators. They also continually improve their capabilities through retraining on new data.
California's fire agency, Cal Fire, uses AI software to analyze images from over 1000 mountaintop cameras across the state. The deep learning models detect visual smoke signatures, notifying firefighters of emerging fires in real-time. During trials, the AI system identified 77 fires before 911 calls, enabling a faster response while the fires were still small.5
However, the technology is constrained to areas visible to cameras and requires human vetting to minimize false alerts.
Pano AI's 5G-Powered Fire Watch
5G technology facilitates faster data transmission and connectivity, enabling the deployment of advanced sensors, drones, and AI for real-time wildfire detection. The high-speed, low-latency capabilities of 5G improve communication among first responders and enhance overall coordination during emergency response efforts.
Pano AI uses ultrahigh definition, 360-degree cameras, and AI on a 5G network to swiftly identify and monitor wildfires. The integration of 5G enhances data transmission and connectivity, allowing for faster response times in remote locations and alerts the nearby public via real-time video transmission.
With over 100 systems deployed in the US and Australia, the technology has detected and aided in the containment of over 50,000 fires since its launch in 2020.6
AI-Integrated Wildfire Detection in Evolving Environments
A study published in the Remote Sensing of Environment proposed a new AI-based model for wildfire detection that combines satellite data with numerical model data used in weather forecasting, enabling a more adaptable and comprehensive approach to monitoring and responding to wildfires.7
The dual-module convolutional neural network (DM CNN) deep learning model evaluates multiple variables simultaneously, including relative humidity, surface temperature, and satellite observation angle. This approach enhances detection accuracy despite changes in environmental conditions, providing a significant advantage over existing technologies.
The technology's wider spatial range compensates for lower satellite resolution, making it a promising advancement in global forest fire detection technology that remains effective despite variations in observing conditions and environmental factors.
Challenges and Future Outlooks
Despite the use of advanced systems like satellites and watchtowers, detecting early-stage wildfires remains challenging. These systems may fail to identify nascent fires promptly, leading to false positives that strain first responder resources.
However, continued innovation across detection modalities and analytics will enable smarter early-warning technology to mitigate escalating wildfire threats.
References and Further Reading
- Mohapatra, A., Trinh, T. (2022). Early Wildfire Detection Technologies in Practice—a Review. Sustainability. doi.org/10.3390/su141912270.
- Min, R. (2023). These Electronic ‘Noses’ Can Sniff Out Wildfires and Alert Fire Authorities Using AI. [Online] Euronews. Available at: https://www.euronews.com/next/2023/07/20/these-electronic-noses-can-sniff-out-wildfires-and-alert-fire-authorities-using-ai.
- Heath, J. (2023). New Drone Research Advances Wildfire Monitoring. [Online] UC Davis. Available at: https://www.ucdavis.edu/climate/news/new-drone-research-advances-wildfire-monitoring.
- Ragbir, P., et al. (2023). UAV-Based Wildland Fire Air Toxics Data Collection and Analysis. Sensors. doi.org/10.3390/s23073561.
- Smith, H. (2023). As California Fires Worsen, Can AI Come to the Rescue? [Online] Los Angeles Times. Available at: https://www.latimes.com/california/story/2023-08-24/can-ai-fight-wildfires-california-is-counting-on-it.
- Sloat, S. (2023). A 5G-Powered Wildfire-Detection Tool Is Helping First Responders Contain Fires Faster. [Online] Business Insider. Available at: https://www.businessinsider.com/pano-ai-5g-wildfire-detection-tool-contains-fires-faster-2023-9.
- Kang, Y., Sung, T., Im, J. (2023). Toward an Adaptable Deep-Learning Model for Satellite-Based Wildfire Monitoring with Consideration of Environmental Conditions. Remote Sensing of Environment. doi.org/10.1016/j.rse.2023.113814.
Disclaimer: The views expressed here are those of the author expressed in their private capacity and do not necessarily represent the views of AZoM.com Limited T/A AZoNetwork the owner and operator of this website. This disclaimer forms part of the Terms and conditions of use of this website.