In a recent article published in the journal Sustainability, researchers focused on applying Unmanned Aerial Vehicle (UAV) hyperspectral imagery to develop new spectral indices (SIs) for predicting the yield of spring maize. The research aims to identify sensitive wavelengths and SIs that can effectively correlate with maize yield across different growth stages and soil types. By leveraging advanced remote sensing technology, the study seeks to provide a technical foundation for improving yield predictions, which is crucial for optimizing fertilizer application and enhancing agricultural productivity.
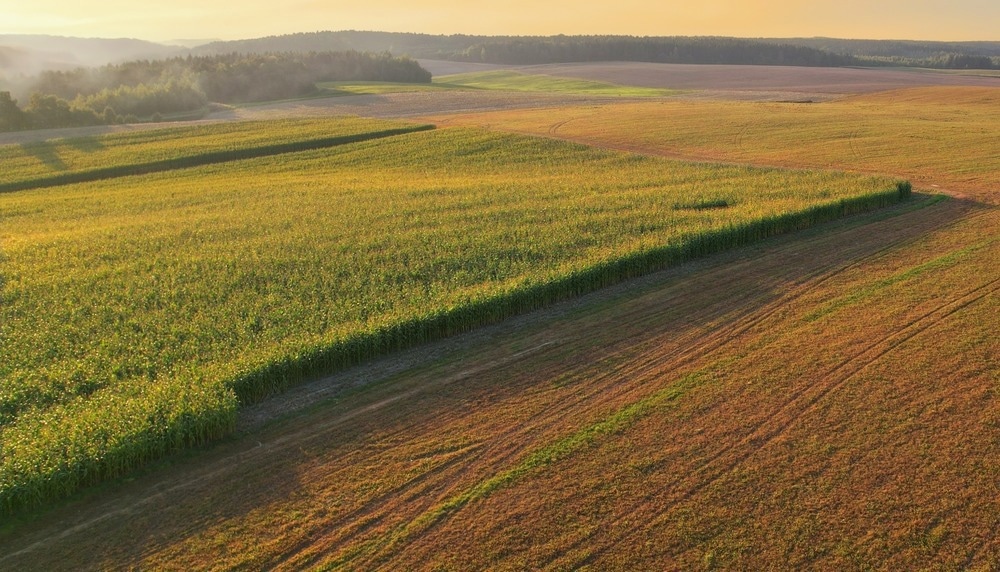
Image Credit: Maksim Safaniuk/Shutterstock.com
Background
The use of hyperspectral imaging in agriculture has gained traction due to its ability to capture a wide range of spectral information, which can be used to assess plant health and predict yields. Traditional methods of yield prediction often rely on limited data and can be influenced by various environmental factors. The study was conducted in three experimental fields located in Lishu County, Jilin Province, China, each characterized by distinct soil types: alluvial soil, black soil, and aeolian sandy soil. These variations in soil properties can significantly affect crop growth and yield. The research builds on previous studies that have explored the relationship between spectral data and crop yield, aiming to refine the predictive capabilities of SIs by identifying new indices that outperform existing ones.
The Current Study
The study's methodology involved several key steps, including selecting the study area and designing field experiments. The experiments were conducted in three sites: Wang-Jia-Qiao (WJQ), San-Ke-Shu (SKS), and Fu-Jia-Jie (FJJ). Each site was divided into microplots, with different nitrogen (N) fertilizer treatments applied to assess their impact on maize yield. The soil properties of each experimental field were meticulously recorded, including sand, silt, clay content, pH, organic matter, total nitrogen, alkali-hydrolyzable nitrogen, available phosphorus, and potassium levels.
UAV hyperspectral images were collected during critical growth stages of maize, specifically focusing on the silking stage, which was identified as the optimal period for yield prediction. The spectral data were processed to calculate various SIs, including newly developed and eight previously published indices. The performance of these indices was evaluated using linear regression analysis to determine their correlation with maize yield. The determination coefficient (R²) was used as a key metric to assess the predictive accuracy of each SI.
The study also involved a comparative analysis of the new SIs against established indices, examining their sensitivity to different N fertilization levels and soil types. This comprehensive approach allowed the researchers to identify the most effective SIs for predicting maize yield under varying conditions.
Results and Discussion
The study's results revealed significant findings regarding the performance of the new spectral indices. Among the newly developed indices, the Normalized Difference Spectral Index (NDSI), the Ratio Spectral Index (RSI), and the Difference Spectral Index (DSI) demonstrated superior predictive capabilities compared to existing indices. The NDSI (690, 710) achieved a determination coefficient of 0.773, the RSI (906, 546) reached 0.892, and the DSI (698, 922) recorded 0.726. These results indicate that the new SIs are more sensitive to variations in maize yield, particularly during the silking stage.
The study also highlighted the distinct responses of the new SIs to different N fertilization levels across the experimental fields, with the exception of the FJJ field. This finding underscores the importance of considering soil type and nutrient management practices when utilizing spectral indices for yield prediction. The ability of the new SIs to accurately reflect yield variations enhances their practical applicability in precision agriculture, allowing farmers to make informed decisions regarding fertilizer application and crop management.
Furthermore, the research contributes to the existing literature by providing a precedent for using UAV-based hyperspectral imagery in agricultural applications. Identifying sensitive wavelengths and effective SIs offers valuable insights for future studies to improve crop yield predictions across diverse climatic regions and farming practices.
Conclusion
In conclusion, this study successfully demonstrated the potential of UAV hyperspectral imagery in developing new spectral indices for predicting the yield of spring maize. The findings emphasize the significance of the silking stage as the optimal period for yield prediction and highlight the superior performance of the newly proposed SIs compared to traditional indices.
The research enhances the accuracy and reliability of yield predictions, providing a valuable tool for optimizing agricultural practices and promoting sustainable farming. Future research is encouraged to further validate the effectiveness of these spectral indices across different crops, climatic conditions, and management strategies, ultimately contributing to the advancement of precision agriculture and food security. The study lays a solid foundation for ongoing exploration in the field, aiming to bridge the gap between technology and practical agricultural applications.
Source:
Zhang Y., Wang Y., et al. (2024). Unmanned Aerial Vehicle (UAV) Hyperspectral Imagery Mining to Identify New Spectral Indices for Predicting the Field-Scale Yield of Spring Maize. Sustainability, 16(24), 10916. DOI: 10.3390/su162410916, https://www.mdpi.com/2071-1050/16/24/10916